“A large fraction of the flaws in software development are due to programmers not fully understanding all the possible states their code may execute in.”
— John Carmack“Can it run Doom?”
— canitrundoom.org
TL;DR
There’s a new entry in my ever-growing vulnerability divination tool suite, designed to assist with reverse engineering and vulnerability research against binary targets: oneiromancer.
It’s a reverse engineering assistant that uses a fine-tuned, locally running LLM to aid with code analysis. It can analyze a function or a smaller code snippet, returning a high-level description of what the code does, a recommended name for the function, and variable renaming suggestions based on the results of the analysis.
As you’ve probably guessed, oneiromancer is written in Rust 🦀 While working on this tool, I also contributed the new security category to crates.io. I hope you’ll find it useful for your projects.
My first official rust-lang contribution 😜https://t.co/SLQ0tnSGXj
On https://t.co/Uo0aEhEXyU, you can now categorize your crates under “security” (“Crates related to cybersecurity, penetration testing, code review, vulnerability research, and reverse engineering.”).
— raptor@infosec.exchange (@0xdea) March 28, 2025
This article is the fourth installment in our Offensive Rust series. In it, I will introduce my new tool and guide you further along your journey into Rust programming. Let’s dive in!
Backstory
Last February, the winners of the annual Hex-Rays Plugin Contest were announced:
We are thrilled to announce the winners of the 2024 Hex-Rays Plugin Contest!
🥇1st Place: hrtng
🥈2nd Place: aiDAPal
🥉3rd Place: idalib Rust bindingsCheck out our reviews of the winners and other notable submissions here: https://t.co/XgkQHfktAF
Huge thank you to all… pic.twitter.com/rw1qzmLjdf— Hex-Rays SA (@HexRaysSA) February 17, 2025
The awesome idalib Rust bindings were awarded a well-deserved third place! But another project caught my attention among the winners: aiDAPal by Atredis Partners. It’s an IDA Pro plugin that uses a locally running LLM that has been fine-tuned for Hex-Rays pseudo-code to assist with code analysis.
Personally, I’m not buying into the current frenzy around vibe reversing and large language models in general. However, I grudgingly have to recognize that there’s more to LLMs than just hype (stay tuned to this blog for some innovative developments in this field that we can’t disclose just yet). Anyway, the premise behind aiDAPal intrigued me, and therefore I decided to test it in the field.
Long story short — I didn’t care much for the plugin’s UX, but I did find the fine-tune of mistral7b-instruct quite impressive. It runs very smoothly under Ollama on my MacBook Air M3 and provides generally actionable output. For the nitty-gritty details behind this custom LLM, I recommend reading How to Train Your Large Language Model by Chris Bellows.
Since the fine-tuned model is freely available on Hugging Face under a permissive license, I thought I could write my own standalone tool to query it. And in my never-ending quest to become a better Rust programmer, I decided to write it in Rust. Really fell in love with this language! I never considered myself a real programmer to begin with (although I’ve always enjoyed coding since I started as a kid with BASIC in the 80s), and I’m definitely no evangelist. I couldn’t care less which language you use 🤷 However, Rust has got something that makes me really want to keep digging deeper and building stuff. But I digress…
Soundtr-hack
“Secret agent man, secret agent man
They’ve given you a number
And taken away your name”
— Johnny Rivers, Secret Agent Man (1966)
Before we look at some Rust learning resources and deep-dive into oneiromancer’s implementation, let’s uphold our tradition by recommending an appropriate soundtrack for your hacking delight! 🕵🏻♂️
Learning resources
Here are some little gems that I recently discovered:
- Updating a Large Codebase to Rust 2024. I found this article useful when upgrading my crates to the Rust 2024 edition. See also Rust Edition 2024 Annotated for a deeper coverage of what’s new in Rust 2024.
- How to Write Documentation. This guide provides clear indications on how to write documentation for a Rust crate, from the basics to more advanced rustdoc features.
- Sentry Rust Development. Another guide that contains a bunch of resources for getting started with Rust and adhering to sound coding principles and engineering practices.
- Setting up effective CI/CD for Rust projects. This short primer demonstrates how to create robust and efficient CI/CD for Rust projects through Github Actions.
- Cargo SemVer Checks. Easily scan your Rust crate for semantic versioning violations, either manually or as part of your CI workflow.
- Pitfalls of Safe Rust. This article showcases a few common gotchas in safe Rust that the compiler doesn’t detect and explains how to avoid them.
- Learn Rust the Dangerous Way. A series of articles that show how to translate an optimized, SSE-using, heap-eschewing C program to Rust, making it immune to crashes and buffer overflows and slightly faster than the original.
- Transition Systems in Rust. This article covers how to implement robust transition systems in Rust, leveraging the language’s powerful type system and ownership model to create maintainable state machines.
- Deploying Rust in Existing Firmware Codebases. Google’s Android Team shows how to gradually introduce Rust into existing firmware, prioritizing new code and the most security-critical code, with drop-in Rust replacements for bare-metal targets.
- No-Panic Rust. This article explores the no-panic technique for systems programming, providing an in-depth discussion of how it works and what problems it solves.
If you’re still struggling with starting your first Rust project, even after perusing the extensive list of learning resources that I’ve shared here and in my past articles, I have a final recommendation for you: The Secrets of Rust: Tools. It’s a recent beginner-friendly book that will guide you through the process of designing command-line tools in Rust, step by step. If you’re stuck, you might want to give it a try.
Querying the Oneiromancer
Oneiromancer
/ō-nī′rə-măn″-sər/
Someone who divines through the interpretation of dreams.
Oneiromancer is a reverse engineering assistant that uses a locally running LLM that has been fine-tuned for Hex-Rays decompiler’s pseudo-code, to aid with code analysis. It can analyze a function or a smaller code snippet, returning a high-level description of what the code does, a recommended name for the function, and variable renaming suggestions based on the results of the analysis. Its main features are:
- Cross-platform support for the fine-tuned LLM aidapal based on mistral7b-instruct.
- Easy integration with my pseudo-code extractor haruspex and popular IDEs.
- Code description, recommended function name, and variable renaming suggestions are printed to the terminal.
- Improved pseudo-code of each analyzed function is saved in a separate file for easy inspection.
- External crates can invoke analyze_code or analyze_file to analyze pseudo-code and then process analysis results.
Additional information on oneiromancer’s features and usage is available at crates.io and in the official documentation.
Let’s install it and take it for a spin! The easiest way to get the latest release is via crates.io:
$ cargo install oneiromancer
Before you can use it, however, you must download and install ollama. Then, download the fine-tuned weights and the Ollama modelfile from Hugging Face:
$ wget https://huggingface.co/AverageBusinessUser/aidapal/resolve/main/aidapal-8k.Q4_K_M.gguf
$ wget https://huggingface.co/AverageBusinessUser/aidapal/resolve/main/aidapal.modelfile
Finally, configure Ollama by running the following commands within the directory in which you downloaded the files:
$ ollama create aidapal -f aidapal.modelfile
$ ollama list
Time to run oneiromancer against a sample pseudo-code file that was previously extracted by haruspex:
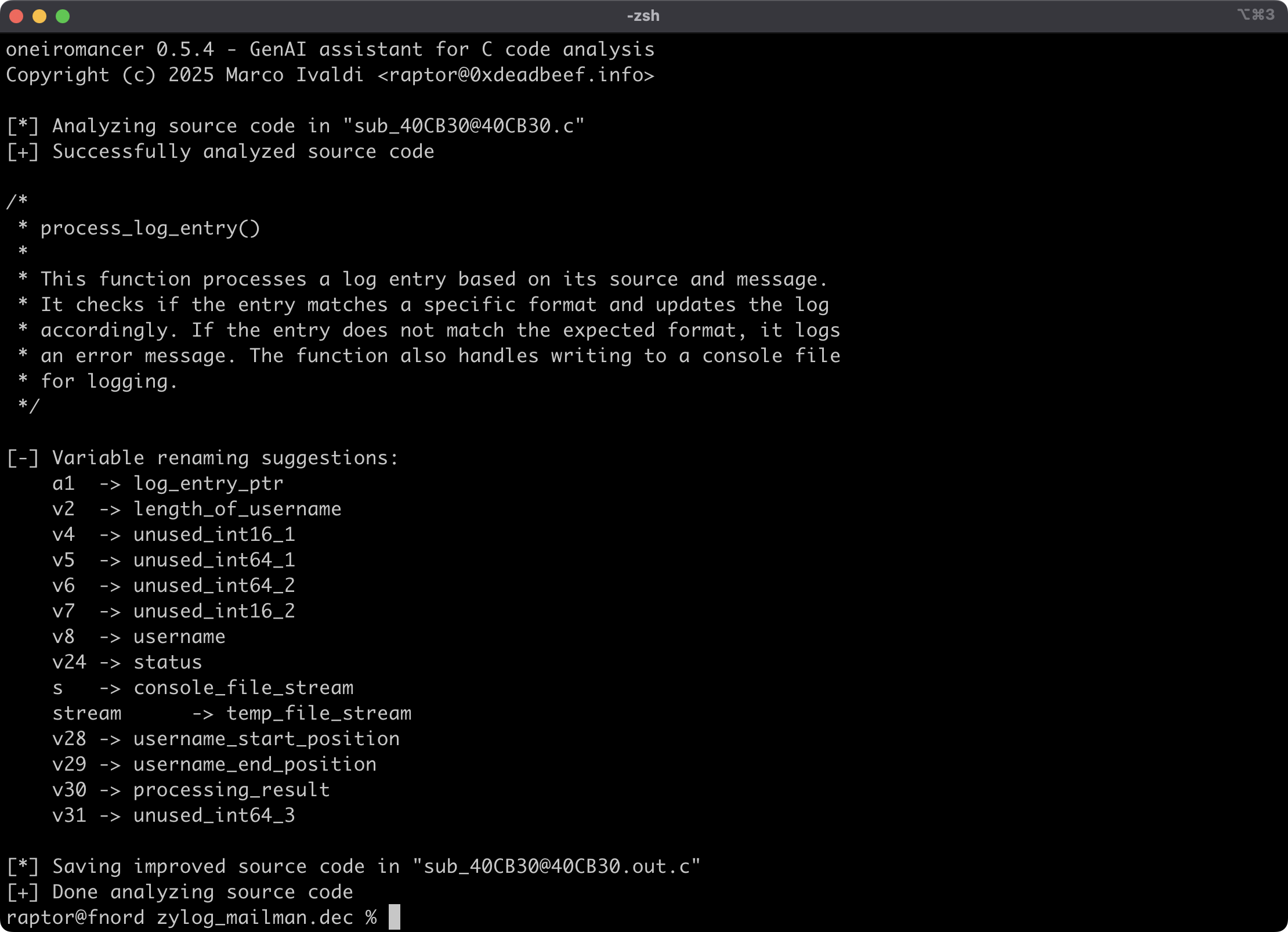
Oneiromancer conveniently saves improved pseudo-code in a separate file:
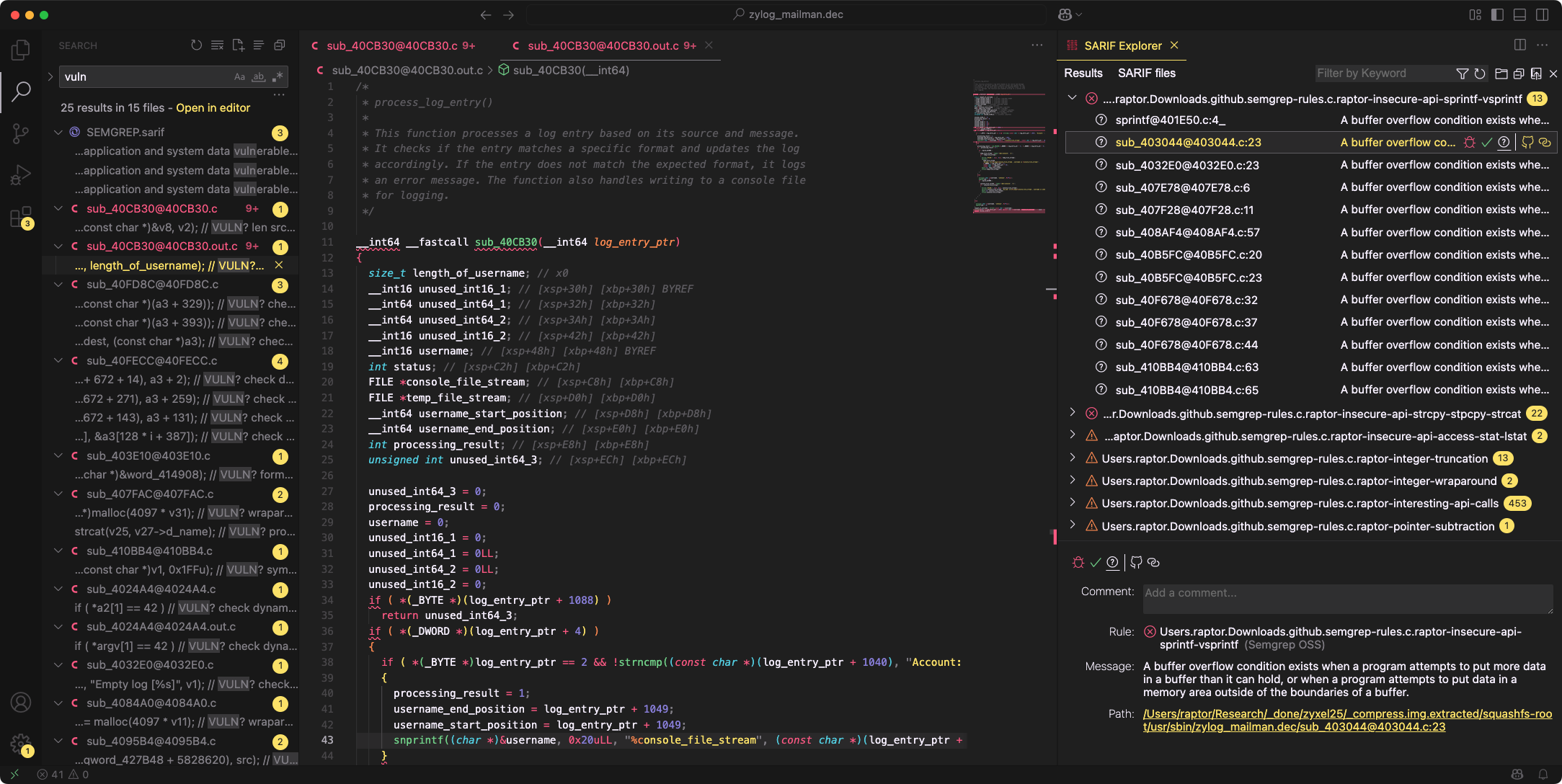
By the way, the above Visual Studio Code screenshot also shows a useful extension that’s relatively new and therefore wasn’t mentioned in my previous article that introduced a simple code review workflow: SARIF Explorer by Trail of Bits. Much better than the alternatives, in my opinion.
The oneiromancer crate can also be easily used as a library in your own crate. Refer to the documentation to see how.
A peek behind the curtain
Let’s take a look at the code now. The core of the oneiromancer crate is actually quite simple. The custom LLM developed by Atredis Partners takes care of the bulk of the work and we just need to query it via the Ollama API.
The run function reads the pseudo-code from the target file at the specified path, submits it to the local LLM for analysis, and parses the results. It then outputs to the terminal a high-level description of what the code does, a recommended name for the function, and variable renaming suggestions based on the results of the analysis. Finally, it saves improved pseudo-code to a separate file:
pub fn run(filepath: &Path) -> anyhow::Result<()> { // Open target source file for reading println!("[*] Analyzing source code in {filepath:?}"); let file = File::open(filepath).with_context(|| format!("Failed to open {filepath:?}"))?; let mut reader = BufReader::new(file); let mut source_code = String::new(); reader .read_to_string(&mut source_code) .with_context(|| format!("Failed to read from {filepath:?}"))?; // Submit source code to local LLM for analysis let mut sp = Spinner::new( Spinners::SimpleDotsScrolling, "Querying the Oneiromancer".into(), ); let analysis_results = analyze_code(&source_code, None, None).context("Failed to analyze source code")?; sp.stop_with_message("[+] Successfully analyzed source code".into()); println!(); // Create function description in Phrack-style, wrapping to 76 columns let options = textwrap::Options::new(76) .initial_indent(" * ") .subsequent_indent(" * "); let function_description = format!( "/*\n * {}()\n *\n{}\n */\n\n", analysis_results.function_name(), textwrap::fill(analysis_results.comment(), &options) ); print!("{function_description}"); // Apply variable renaming suggestions println!("[-] Variable renaming suggestions:"); for variable in analysis_results.variables() { let original_name = variable.original_name(); let new_name = variable.new_name(); println!(" {original_name}\t-> {new_name}"); let re = Regex::new(&format!(r"\b{original_name}\b")).context("Failed to compile regex")?; source_code = re.replace_all(&source_code, new_name).into(); } // Save improved source code to output file let outfilepath = filepath.with_extension("out.c"); println!(); println!("[*] Saving improved source code in {outfilepath:?}"); let mut writer = BufWriter::new( File::create_new(&outfilepath) .with_context(|| format!("Failed to create {outfilepath:?}"))?, ); writer.write_all(function_description.as_bytes())?; writer.write_all(source_code.as_bytes())?; writer.flush()?; println!("[+] Done analyzing source code"); Ok(()) }
The following functions, that can also be called by external crates, are in charge of submitting pseudo-code (either directly or contained in a file) to the local LLM for analysis. If needed, you can specify custom OLLAMA_BASEURL and OLLAMA_MODEL values via the environment.
pub fn analyze_code( source_code: &str, baseurl: Option<&str>, model: Option<&str>, ) -> Result<OneiromancerResults, OneiromancerError> { // Check environment variables let env_baseurl = env::var("OLLAMA_BASEURL"); let env_model = env::var("OLLAMA_MODEL"); // Send Ollama API request and parse response let request = OllamaRequest::new( model.unwrap_or_else(|| env_model.as_deref().unwrap_or(OLLAMA_MODEL)), source_code, ); request .send(baseurl.unwrap_or_else(|| env_baseurl.as_deref().unwrap_or(OLLAMA_BASEURL)))? .parse() } pub fn analyze_file( filepath: impl AsRef<Path>, baseurl: Option<&str>, model: Option<&str>, ) -> Result<OneiromancerResults, OneiromancerError> { // Open target source file for reading let file = File::open(&filepath)?; let mut reader = BufReader::new(file); let mut source_code = String::new(); reader.read_to_string(&mut source_code)?; // Analyze `source_code` analyze_code(&source_code, baseurl, model) }
The analyze_code and analyze_file functions use the OllamaRequest, OllamaResponse, OneiromancerResults, and OneiromancerError abstractions defined in separate files.
For further details, refer to oneiromancer’s source code on GitHub:
Acknowledgments
Once again, the heavy lifting of fine-tuning the local LLM to analyze pseudo-code generated by the Hex-Rays decompiler was done by Chris Bellows and Atredis Partners. They deserve all credit for training the aidapal custom LLM that’s queried by oneiromancer.